Update
December 9, 2024
TZ-SAM: A brighter spotlight on global solar growth
Improvements to Solar Asset Mapper’s underlying machine learning technology make it more reliable, more accurate and faster to run

A major update
Launched in May 2024 in collaboration with Global Energy Monitor, TransitionZero’s open-access Solar Asset Mapper (TZ-SAM) dataset has become an essential research tool for hundreds of power analysts worldwide.
Following user feedback - and a generous research grant from the Banyan Software Foundation - we are thrilled to share a new release of TZ-SAM.
The Q3 2024 release of TZ-SAM boasts significant improvements to methodology, accuracy and scope.
In this update, we'll look at these improvements, discuss how they can support energy transition analysts, and share our research roadmap.
Why do we need TZ-SAM?
Global solar PV deployment continues on a sharp, upward trajectory.
Accurate data is critical for effective energy planning, policy design, and investment decision-making. Yet the rapid, decentralised nature of solar PV growth makes it difficult to get a clear snapshot.
The official data is rubbish. I cannot tell you how uncertain we are about how much solar is actually out there.

Jenny Chase, Lead Solar Analyst
BloombergNEF
TZ-SAM addresses this need by providing detailed, open-access, asset-level data for over 81,000 solar facilities in 190 countries. It includes the location, capacity, size, and estimated construction dates of these installations. This dataset captures small-scale utility installations (1–5MW) and larger facilities, filling a critical gap in traditional, public datasets.
Solar is the only renewable energy source on track to meet 2030 targets, growing at a phenomenal rate. Up-to-date, accurate and accessible solar PV data is crucial to support effective energy planning decision-making.

Joe O’Connor, Head of Machine Learning
TransitionZero
Today, over 500 analysts from banks, governments, and consultancies use TZ-SAM data to forecast trends, model grid demand, and design energy policy. It’s become a go-to resource for data journalists at prestigious outlets like The Economist and The New York Times.
Learn more about TZ-SAM’s scientific foundation, and how our machine learning research team were able to scale this research, in our TZ-SAM Explainer, or watch our webinar, ‘How planetary-scale machine learning improves data quality for energy transition planning’.
So, what have we improved exactly?
TZ-SAM gets a tune up
TZ-SAM Q3 ‘24 tracks 853GW of solar capacity, a staggering 21% increase on our first release.
But scale is irrelevant if the data cannot be trusted. Revisions to the underlying algorithms have cut our detection error rate in half, from 1% to less than 0.5%
An additional 21,350 square kilometers of solar - over 18,000 individual facilities - have been added to the database, with eight more countries now tracked by TZ-SAM.
While the dataset includes industrial- and utility-scale solar farms, TZ-SAM excels at detecting small-scale solar - assets with less than 5MW capacity - plugging significant data gaps for dispatch modelling and grid-level analysis.
Our algorithm now detects smaller solar facilities with even greater precision, and can better distinguish novel detections from structures like parking lots, greenhouses or bodies of water. Capacity estimation methods have also improved, enabling more accurate projections of solar’s contribution to grids. Analysts can now better account for smaller, distributed solar systems, which continue to play a growing role in the energy transition.
Together, these improvements support asset and regional level analysis, particularly in overlooked developing markets, remote areas, or in controlled markets where public data may be unreliable.
Quarterly releases
Automation is just one major benefit of using machine learning for data collection and validation.
Today, the detection-to-data pipeline is nearly fully automated, enabling quarterly updates to begin in 2025. Analysts can build TZ-SAM into their workflows, and will benefit from reliable, regular access to a global snapshot of this booming sector. We hope this quarterly release cadence will help TZ-SAM users generate more precise forecasts and trustworthy analysis.
What’s next?
Improvements to TZ-SAM are funded by a $1M grant from the Banyan Software Foundation. This funding supports ongoing development, and allows us to distribute TZ-SAM as a public good for non-commercial use. We will continue to make improvements to TZ-SAM metadata and to our capacity estimation algorithms, while adding quality-of-life improvements to our browser UI.
However, we’re particularly excited about our research into residential rooftop solar.
It's a popular feature request from early TZ-SAM users, which comes as no surprise: Australia’s Clean Energy Council estimates that rooftop solar now accounts for 11% of the country’s power supply.
This research is moving at pace. A test case running in the Philippines, a focus country for TransitionZero’s in-market analysts, shows promising early results. A limiting factor for this work is access to sufficiently high-resolution photography and ‘ground truth’ capacity data for model calibration.
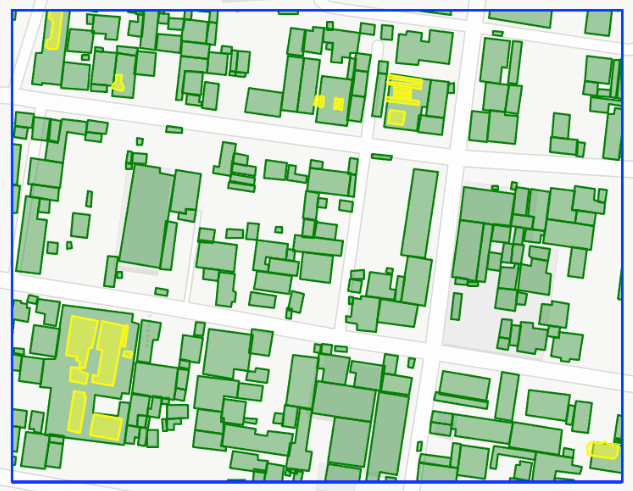
Image: TZ-SAM’s ability to accurately detect rooftop solar in the Philippines, using high-resolution imagery from ESRI.
Download TZ-SAM
The TZ-SAM Q3 2024 dataset is open-access under a Creative Commons license for non-commercial use. Download it directly from our website now, and sign up for notifications to our quarterly updates beginning in 2025.